 |

Vowels |
a, i, u, e, o (voiced), A, I, U, E, o (devoiced), aH, iH, uH, eH, oH (long vowels) |
Plain consonants |
k, g, G[Υ], @[ ], s, z, t, c[ts], d, n, h, F, b, p, m, r, w |
Phonetically palatalized (before /I/) consonants |
kj, gj, Gj, @j, sj[ ], zj[ ], cj[t], nj[ ],hj[Ç], |
Phonologically palatalized consonants |
ky, gy, Gy, @y, sy, zy, cy, ny, hy, ny, hy, by, py, my, ry |
Special morae |
N (moraic nasal), Q (geminate), H(long vowel) |
Special labels |
#, <cl>, <pz>, <uv>, <sv> , <fv>, <?>, <N>, <b> |
|
This table shows the inventory of the segmental labels used for the labeling of the CSJ-Core. The labels are basically phonemic, but there are some phonetic labels, too. Devoiced vowels, phonetic palatalization, and, variants of /g/ phoneme are the examples. Special labels are used to refe r to sub-phonemic events like closure of stops and affricates (<cl>), utterance-internal pause (<pz>), succession of voicing after the end of an utterance (<uv>), and so forth.
This inventory was designed to cover segmental phonological variations observed in Tokyo Japanese, but some of the labels are not used in the labeling of the CSJ-Core. Currently, we do not make distinction between '@' and'g', because there was considerable inter- labeler disagreement.
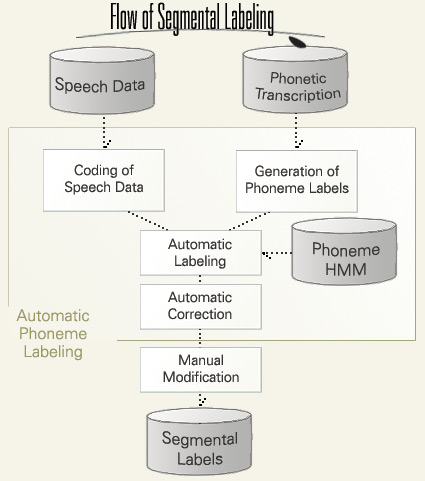
The process of segmental labeling was half- automated by use of the HMM-based speech recognition technique. This figure shows the flowchart of segmental labeling. Phoneme label sequence was generated from the ("phonetic") transcription. The generated phoneme labels are aligned to speech signal using phoneme HMM. Some of the phoneme labels are transformed into phonetic ones by rule. Human labelers, then, inspect the aligned labels and correct them if necessary.
At last, human labeler will modify the them manually. Automatic label generation and alignment reduced the time of labeling to about 50%.
Here is an example of segmental labels. The sample material is "watashi wa ryokoH ga daisuki de" (I like traveling very much).
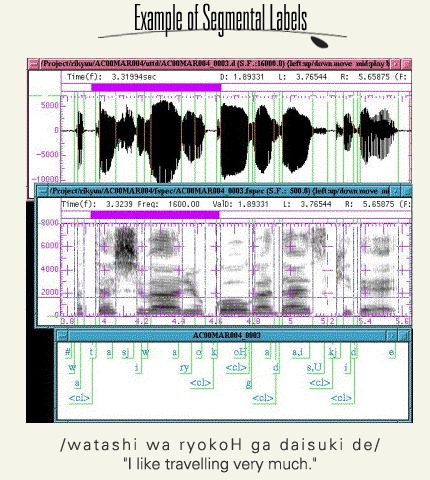
In order to evaluate the accuracy of segmental labeling, inter- labeler reliability was evaluated. Difference of label boundary was measured as the index of inter- labeler agreement.
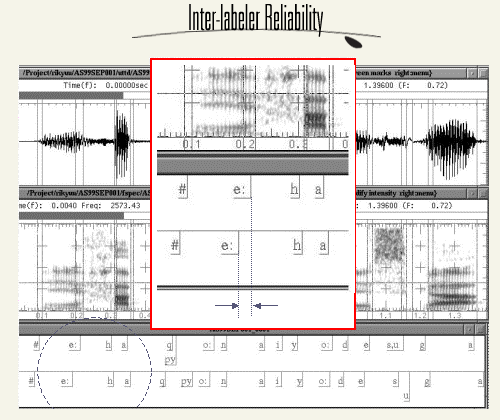
Sample |
Labeler A |
Labeler B |
Diff. (ms) |
# |
time[s] |
total |
merged |
total |
merged |
(Abs.) |
1 |
22.4 |
298 |
29 |
282 |
36 |
7.78 |
2 |
24.2 |
348 |
30 |
299 |
36 |
7.76 |
3 |
22.9 |
312 |
31 |
291 |
41 |
7.70 |
4 |
33.6 |
408 |
25 |
378 |
45 |
8.34 |
5 |
22.2 |
275 |
19 |
261 |
25 |
6.76 |
6 |
32.0 |
396 |
33 |
350 |
56 |
11.46 |
7 |
19.2 |
253 |
17 |
237 |
26 |
8.37 |
8 |
28.3 |
307 |
24 |
306 |
26 |
9.73 |
9 |
23.0 |
301 |
22 |
275 |
35 |
7.03 |
whole |
227.8 |
2898 |
230 |
2679 |
326 |
8.37 |
rate [%] |
- |
7.9 |
- |
12.2 |
|
This table compares the labeling results of two expert labelers who labeled 9 short samples excerpted from CSJ. The mean of absolute difference is about 8 ms.
Compared to the mean difference of ATR speech database, which deals with the material of word list reading, this is not a bad performance as the labeling of spontaneous speech material.
Lastly, accuracy of automatic labeling (without human correction) was evaluated. For this purpose, two HMM phoneme models were applied for three types, namely, read, spontaneous monologue, and spontaneous dialogue.
The first HMM model was trained with 40 hours of read speech taken from the JNAS corpus, which contains the reading of "Mainichi" newspaper articles.The second one was trained with 59 hours of the CSJ. The condition of acoustic analysis is shown in the bottom of the slide. |
 |
Acoustic model : HMM phoneme model |
Training data : |
Reed speach |
JNAS |
40[h] |
Spontaneous speech |
CSJ |
59[h] |
Condition of acoustic analysis |
Sampling rate |
16[kHz] |
Frame lengh |
25[ms] |
|
Frame Shift |
10[ms] |
|
Feature parameter |
12MFCC+12ΔMFCC+ΔPower |
|

Target data |
Std. Dev. Of Diff.[ms] |
Acoustic model |
ATR-DB |
18.63 |
JNAS-mono |
APS of CSJ |
20.50 |
JNAS-mono |
SPS of CSJ |
21.60 |
JNAS-mono |
All of CSJ |
21.72 |
CSJ-mono |
Dialogue([Osuga 2001]) |
29.4 |
JNAS-mono |
Dialogue([Mera 2001]) |
28.19 |
JNAS-mono |
Dialogue([Wightman 95]) |
22.7 |
monophone |
|
Difficulty of segmental labeling : Read < CSJ < Dialogue
Training Data : Read > CSJ
This table shows that the performance of automatic labeling applied for CSJ spontaneous speech is intermediate between the performance of read speech data (ATR database) and spontaneous dialogue data (reported by Osuga, Mera, and Wightman). Among the CSJ samples, SPS was more difficult than APS.
It is also interesting to see that the performance of acoustic model trained by CSJ data was not better than the performance of the model trained by JNAS data.
Presumably, this is because the acoustic boundaries in the read speech data are clearer than that of spontaneous speech, and, accordingly, the read speech model could detect the boundary features more accurately than the spontaneous speech model.
|